In the subsurface, the natural porous medium has a complex topology, making two-phase displacement simulations challenging; as a result, researchers have proposed various models to simulate this by interpreting key features of capillary pressure measurements. However, only a few models map pore-scale processes (~10 nm–1 μm) to centimeter-scale behavior, and most require parameter tuning. More importantly, these models depend on researchers’ interpretation of the measurements. This research aims to interpret capillary pressure using machine learning.
Machine Learning for Capillary Pressure Estimation
The Leverett J function provides a convenient tool for estimating capillary pressure in the subsurface; however, it performs poorly when its results are scattered. This study used pattern-recognition algorithms to enhance performance by analyzing samples from North America and the Middle East. First, it formulated the features (F1 and F2, as discussed in Kasha et al. 2022) based on permeability and porosity. It then clustered the samples and applied four classification algorithms: kernel support vector machine (KSVM), k-nearest neighbors (KNN), decision tree (DT), and random forest (RF). There was no need for data augmentation.

This study assessed performance by determining the ratio of the J function (Jmax(Sw)/ Jmin(Sw)). The proposed approach yields a much smaller ratio than the common method, indicating that the scatter has reduced significantly. Additionally, the proposed approach performs better than the flow zone indicator (FZI) when the number of clusters is reasonable (<10).
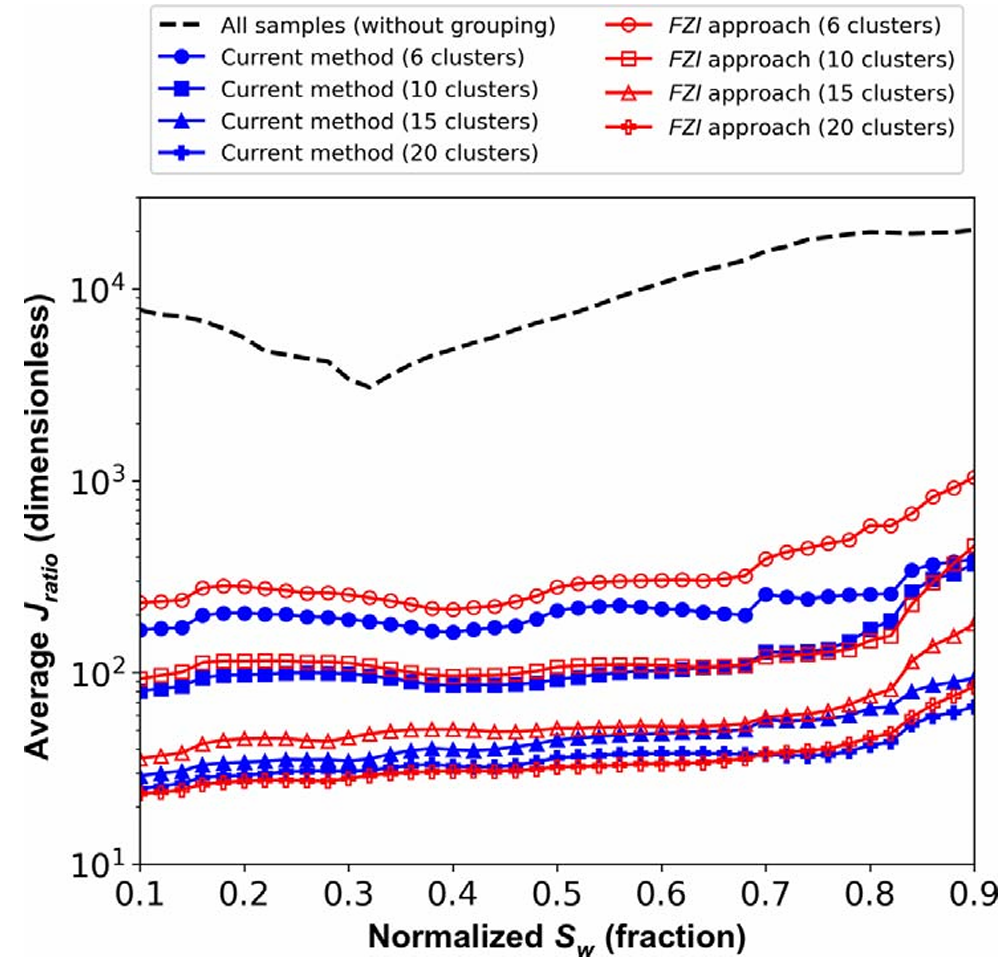
Predicting Permeability from Capillary Pressure Using Machine Learning
Capillary pressure measurements are used to characterize formation permeability from small samples when large samples, such as core plugs, are unavailable. This study employed machine learning to predict carbonate formation permeability by analyzing the properties of 193 carbonate samples (porosity, permeability, and mercury injection capillary pressure.) The permeability values varied from nanodarcies to darcies. This study proposed two new correlations for permeability prediction, both with and without grouping. The results are promising, with the average R2 exceeding 0.96.

Representative articles
- Kasha, A., Sakhaee-Pour, A., and Hussein, I. (2022). Machine Learning for Capillary Pressure Estimation. SPE Reservoir Evaluation & Engineering, 25 (01): 1–20.
- Tran, H., Kasha, A., Sakhaee-Pour, A., and Hussein, I. (2020). Predicting carbonate formation permeability using machine learning. Journal of Petroleum Science and Engineering, 195, 107581.